Predicting the Next Overdose (022)
Study Information
Predictive analytics is a powerful tool to determine the risk of fatal and nonfatal overdose and re-arrest and re-offending of criminal justice-involved individuals living with opioid use disorder.
The University of Chicago is developing open-source software that can be used by researchers and practitioners to predict overdose and re-offending risk of their population. This project will use large administrative datasets and machine-learning technology to develop a framework for transparent predictive models and simulations to help identify people at highest risk for overdose and/or re-offending and how populations will benefit from interventions, and explore the likely policy impact of observed relationships among emerging trends to improve outcomes.
Study Team
PI: Harold Pollack, PhD
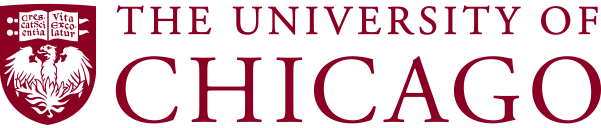
• Create a linked administrative dataset of first response, criminal justice, and treatment system data, with identified features associated with overdose and re-offending risk
• Implement machine-learning analysis for identified samples of individuals and locations
• Create open-source software to share with researchers and practitioners throughout JCOIN
Modeling Project